Imaging offers usuful insights in pathologies of the lung. Many of our studies deal and have dealt with improving the extraction of information that is provided in this valueable information source.
Projects
Ongoing: ONSET; Finished: BIGMEDILYTICS. KHRESMOI, PULMARCH
Highlight Publications
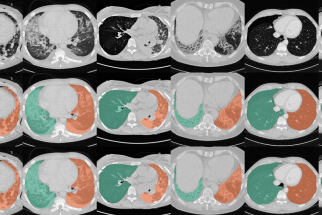
Segmentation Automated segmentation of anatomical structures is a crucial step in many medical image analysis tasks. We show that a basic approach - U-net - performs either better, or competitively with other approaches on both routine data and published data sets, and outperforms published approaches once trained on a diverse data set covering multiple diseases. Training data composition consistently has a bigger impact than algorithm choice on accuracy across test data sets. (Hofmanninger et al, 2020)
The code for lung segmentation is available: code releases
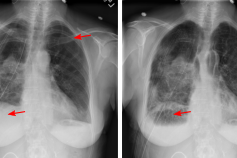
X-ray X-ray is a commonly used low-cost exam for screening and diagnosis. However, X-ray radiographs are 2D representations of 3D structures causing considerable clutter impeding visual inspection and automated image analysis. Here, we propose a Fully Convolutional Network to suppress undesired visual structure from radiographs while retaining the relevant image information such as lung-parenchyma. (Hofmanninger et al. 2019)
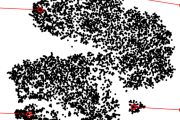
Phenotyping A key question in learning from clinical routine imaging data is whether we can identify coherent patterns that re-occur across a population, and at the same time are linked to clinically relevant patientparameters. Here, we present a feature learning and clustering approach that groups 3D imaging data based on visual features at corresponding anatomical regions extracted from clinical routine imaging data without any supervision. (Hofmanninger et al, 2016)
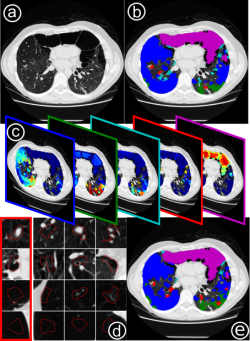
Weakly-supervised learning for mapping visual features to semantic profiles To learn models that capture the relationship between semantic clinical information and image elements at scale, we have to rely on data generated during clinical routine (images and radiology reports), since expert annotation is prohibitively costly. Here, we show that re-mapping visual features extracted from medical imaging data based on weak labels that can be found in corresponding radiology reports creates descriptions of local image content that captures clinically relevant information. In medical imaging (a) only a small part of the information captured by visual features relates to relevant clinical information such as diseased tissue types (b). However, this information is typically only available as sets of reported observations on the image level. Here, we demonstrate how to link visual features to semantic labels (c), in order to improve retrieval (d) and map these labels back to image regions (e). (Hofmanninger and Langs, 2015)
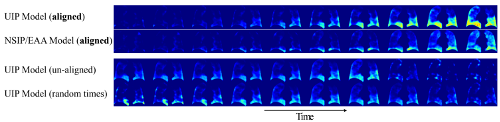
Fibrosis Generating disease progression models from longitudinal medical imaging data is a challenging task due to the varying and often unknown state and speed of disease progression at the time of data acquisition, the limited number of scans and varying scanning intervals. We propose a method for temporally aligning imaging data from multiple patients driven by disease appearance. It aligns followup series of different patients in time, and creates a cross-sectional spatio-temporal disease pattern distribution model. (Vogl et al, 2014)
Publications
- Röhrich, S., Hofmanninger, J., Negrin, L., Langs, G. and Prosch, H.: Radiomics score predicts acute respiratory distress syndrome based on the initial CT scan after trauma. European Radiology (2021), pp.1-11.
- Johannes Hofmanninger, Forian Prayer, Jeanny Pan, Sebastian Röhrich, Helmut Prosch and Georg Langs: Automatic lung segmentation in routine imaging is primarily a data diversity problem, not a methodology problem. Eur Radiol Exp (2020) 4, 50. https://doi.org/10.1186/s41747-020-00173-2
- F. Prayer, J. Hofmanninger, M. Weber, D. Kifjak, A. Willenpart, J. Pan, Se. Röhrich, G. Langs, H. Prosch: Variability of computed tomography radiomics features of fibrosing interstitial lung disease: A test-retest study. Methods (2020)
- Röhrich, S., Hofmanninger, J., Prayer, F., Müller, H., Prosch, H. and Langs, G.: Prospects and Challenges of Radiomics by Using Nononcologic Routine Chest CT. Radiology: Cardiothoracic Imaging (2020), 2(4), p.e190190.
- Röhrich, S., Schlegl, T., Bardach, C., Prosch, H. and Langs, G.: Deep learning detection and quantification of pneumothorax in heterogeneous routine chest computed tomography. European Radiology Experimental (2020), 4, pp.1-11
- Johannes Hofmanninger, Sebastian Roehrich, Helmut Prosch and Georg Langs: Separation of target anatomical structure and occlusions in thoracic X-ray images, 33rd Conference on Neural Information Processing Systems (NeurIPS 2019), https://arxiv.org/abs/2002.00751
- Johannes Hofmanninger, Markus Krenn, Markus Holzer, Thomas Schlegl, Helmut Prosch, and Georg Langs: Unsupervised Identification of Clinically Relevant Clusters in Routine Imaging Data. International Conference on Medical Image Computing and Computer-Assisted Intervention (MICCAI 2016)
- Johannes Hofmanninger, and Georg Langs: Mapping Visual Features to Semantic Profiles for Retrieval in Medical Imaging. Proceedings of the IEEE Conference on Computer Vision and Pattern Recognition (CVPR 2015), Code: https://github.com/JoHof/semantic-profiles
- Wolf-Dieter Vogl, Helmut Prosch, Christina Mueller-Mang, Ursula Schmidt-Erfurth, and Georg Langs: Longitudinal Alignment of Disease Progression in Fibrosing Interstitial Lung Disease. International Conference on Medical Image Computing and Computer-Assisted Intervention (MICCAI 2014)
Images: MUW/Hofmanninger, Hofmanninger, Hofmanninger, Hofmanninger, Vogl