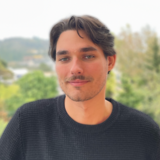
Simon Gutwein
Research Associate and PhD CandidateContact
Email: simon.gutwein@ccri.at
Computational Imaging Research Lab
Early Life Image Analysis Group
Department of Biomedical Imaging and Image-guided Therapy
Medical University of Vienna
Währinger Gärtel 18-20
1090 Vienna / Austria
St. Anna Children’s Cancer Research Institute
Taschner Mandl Lab
Zimmermannplatz 10
1090 Vienna / Austria
Simon Gutwein is a research associate at the ELIA Group at CIR and pursuing his PhD at the Computer Vision Lab at TU Wien in collaboration with the Taschner-Mandl Group at the Children Cancer Research Institute (CCRI) and ELIA Group at CIR.
He obtained his Bachelor's in Biomedical Engineering from the University of Applied Sciences Saar and graduated the Master study of Medical Radiation Sciences at the University of Tübingen.
His research interest lies in computational biology and deep learning applications, with a focus on analyzing high-dimensional imaging data from advanced techniques like Imaging Mass Cytometry (IMC) and Fluorescence in Situ Hybridization (FISH). His work aims to address the critical challenges in biological image analysis by developing scalable, interpretable, and efficient computational frameworks. He is passionate about using machine learning to unlock new insights into cell-type heterogeneity, spatial organization, and genetic aberrations in complex tissues.
Since May 2024 he is a visiting research fellow at the PSL University, Institut Curie and INSERM, in Paris, France under the supervision of Thomas Walter. Link
Research interests
- Exploring cell types and tumor imaging
- Leveraging AI to revolutionize medical diagnostics and treatments
- Investigating uncertainties in deep learning for reliable AI in healthcare
Awards
-
Best Poster Presentation Award of the UNSURE Workshop held in conjunction with MICCAI 2024 in Marrakech Marokko, October 2024.
-
Best Student Paper Award Nomination, International Conference Pattern Recognition Applications and Methods (ICPRAM), Rome, February 2024.
Projects
- Segmentation-Free Analysis of Imaging Mass Cytometry Data
- GENUINE: Genetic Aberration Classification in FISH Images
Recent Publications
Gutwein, S.; Lazic D.; Walter T.; Taschner-Mandl S.; Licandro R., Interpretable Embeddings for Segmentationn-free Single-Cell Analysis in Multiplex Imaging, submitted, https://doi.org/10.48550/arXiv.2411.03341, November 2024.
Gutwein, S.; Kampel, M.; Taschner-Mandl, S.; Licandro, R.,FISHing in Uncertainty: Synthetic Contrastive Learning for Genetic Aberration Detection. In: Uncertainty for Safe Utilization of Machine Learning in Medical Imaging. MICCAI-UNSURE 2024. Lecture Notes in Computer Science, vol 15167. Springer, Cham. https://doi.org/10.1007/978-3-031-73158-7_3 openreview, Best Poster Presentation Award, October 2024.
Gutwein, S.; Kampel, M.; Taschner-Mandl, S. and Licandro, R., GENUINE: Genomic and Nucleus Information Embedding for Single Cell Genetic Alteration Classification in Microscopic Images. In Proceedings of the 13th International Conference on Pattern Recognition Applications and Methods - ICPRAM; ISBN 978-989-758-684-2; ISSN 2184-4313, SciTePress, pages 27-36. DOI: 10.5220/0012319700003654. February 2024